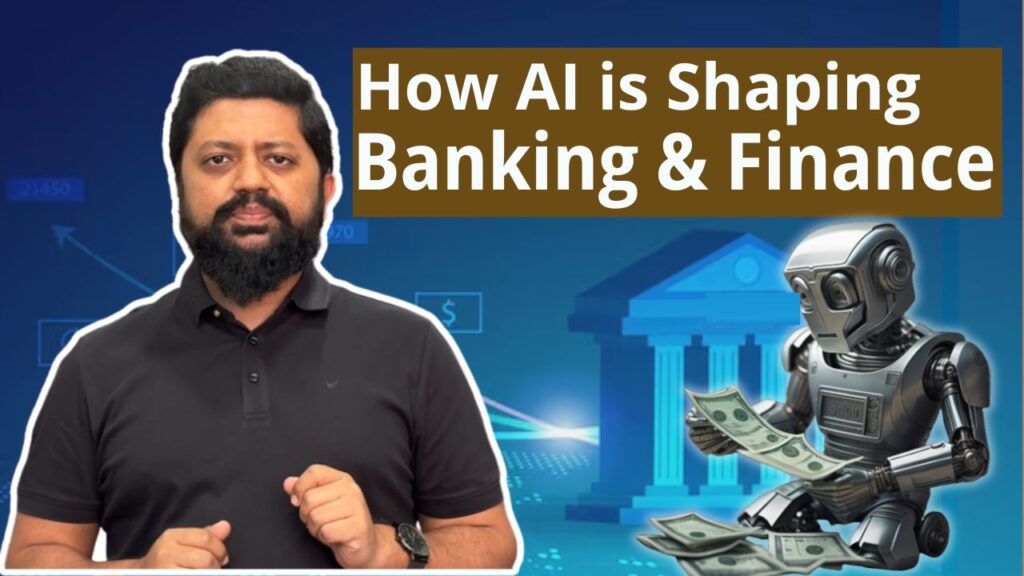
Did you know that by 2030, the global AI market in the banking sector is projected to reach over $64 billion? Prominent figures like the former Citigroup chief Vikram Pandit have predicted back in 2018 that substantial portions of banking jobs, ranging from 30% to even 50 and 70%, could be replaced by AI in the next few years. So, has it happened already? From analyzing contracts in seconds to emojis helping you manage your finances, we have got some mind blowing innovations to talk about. So feel free to share this article with your team on LinkedIn.
JP Morgan – Contract Intelligence
Picture the verbose and arguably boring world of legal contracts and agreements. It’s time consuming, error prone and frankly, a bit tedious. But what if we could change that? What if we could automate this task of contract reviewing? Well, hold on to your hats, because JP Morgan has done just that.
JP Morgan Chase is the largest bank in the United States and the world’s largest bank by market cap. They have introduced something called contract intelligence, or COiN for short. And COiN isn’t your mom’s legal software. It’s armed with machine learning and natural language processing, or NLP. It can analyze complex legal documents and extract crucial information in a fraction of the time it takes for a human to do the same thing. JP Morgan says that lawyers spent an upward of jaw dropping 360,000 hours every year deciphering commercial credit agreements.This was, of course, before COiN came along, and that’s not it. This technology operates on a private cloud network called Gaia, which also was developed in house by JP Morgan Chase.
How does it work? It employs something called an unsupervised machine learning process, which means after it has been set up with the algorithms and fed with the annotated training data, you know, the kind of work that we do at Esycommerce, there’s very little need for humans to be involved. The essential technique used here to compare and distinguish between different agreements is called optical character recognition, or OCR. Based on the training datasets of historical contracts, and by recognizing patterns in words and their locations in the contracts, COiN identifies clauses, terms, legal language, and their repercussions. It can even spot discrepancies and anomalies within contracts, streamlining legal processes for a more focused and efficient approach. But that’s just the tip of the iceberg.
JP Morgan is all in on AI apparently. They’ve set up tech centers dedicated to big data, robotics and cloud infrastructure. Zames Matt, the former chief operating officer over at JPM, mentioned how this system is giving them and their developers a major boost in productivity. And the cherry on top? It’s actually saving them a good chunk of capital.
The bank has involved the best AI talents around the globe in the last few years. They have assembled the likes of Manuela Veloso, who has been the head of AI research at JPM since the July of 2018, while Dr. Samina Shah operates as the managing director in the AI research organization at JPMorgan. Then there’s Tucker Balch, a respected AI researcher from Georgia Tech who was hired as the managing director of AI research in 2019. With hiring moves like these, JPM is making it clear that this isn’t just a phase, it’s a financial AI revolution.
PayPal- Fraud Detection
Ever tried giving money to a distressed Nigerian prince? Don’t worry, because apparently you’re not alone. As digital transactions soar, so does the risk of cybercrime. But here’s where AI steps in, and PayPal is leading the charge. PayPal, which once pioneered digital payments, has taken proactive steps to safeguard its customers.
Over the last few years, they have put a lot of effort into artificial intelligence and data analytics to make sure that they can handle risks better to keep their customers safe. In 2018, PayPal acquired Simility, a fraud detection startup, and this move allowed them to strengthen their risk assessment and fraud prevention capabilities. After PayPal got their hands on Simility, Bill Ready, PayPal’s then chief operating officer pointed out how PayPal solutions used to have a one size fits all approach. They wouldn’t always fit with the specific needs of different merchants out there. But now, with Simility in the picture, it’s like a whole new ballgame.
PayPal can actually give merchants more power to take charge and tackle fraudulent transactions head on. They have integrated fraud protection into PayPal commerce platform. This integration comes with a user friendly dashboard interface. The platform provides tailored fraud filters based on each merchant’s specific business data that they generate. Once they start using the platform, merchants can also assess the effects of these filters on past data before putting them into action.
The system constantly offers suggestion for improvement to enhance the effectiveness of these filters. The best part is that they are not reacting to fraud they are predicting and preventing it. Their secrete sauce! Graph Analysis. You see graph analysis is like putting together a puzzle. But this puzzle reveals the connections between buyers and sellers and their extended networks as well the connection in these payment networks can strongly predict behaviors and serve as excellent signs of risk thus detecting potential fraud.
However, as PayPal claims, these relationships are too huge to traverse and analyze if they were to be stored in a relational database. The company uses something called a graph analysis. It allows PayPal to create a payments graph that shows the transactions between buyers and sellers. Through graph analysis, PayPal can identify unauthorized access gained by a user account. By examining the connections and the patterns in the graph, it can predict whether a buyer is likely to engage with a seller that is deemed risky based on their existing connections and historical patterns within the graph. It can also forecast future interaction through graph analysis. It’s like having an AI Sherlock Homes detecting fraud before it even happens. There are two types of graph analysis at play discrete and connected.
Discrete graph Analysis: It focuses on individual items that could be buyers, sellers, transactions and other related elements. The goal is to assess the risk associated with each entity by analyzing their associations and connections. For example, consider a buyer with a history of fraud or suspicious activity. If this buyer frequently interacts with another specific seller, the algorithm may examine that behavior and can flag that buyer as potentially risky based on his negative history.
Connected Graph Analysis: It zooms out to see the bigger picture. It’s like solving a mystery by connecting the dots. This type of analysis considers how entities are interconnected and how the graph as a whole functions. Various graph algorithms are used to extract meaningful information from graph’s interconnected data. By applying various graph algorithms like Page rank connected components, clustering centrality algorithms, analysts can uncover insights such as influential sellers, distinct communities of users, and potential ring frauds as well. For example, a group of fraudsters operating from the same IP address could raise alarms for all transactions originating from similar ranges of IP’s in the same geographical vicinity.
You see, PayPal’s proactive approach isn’t about safeguarding your money, it’s about gaining your trust. And guess what? They are the first foreign payment platform approved for online services in China, a country that finds extremely hard to trust anything that isn’t born out of China itself. Impressive, Right!
Capital One: ENO
Now, let’s switch gear to customer interactions and chatbots. We have all encountered them, but Capital one, a US based financial corporation, took it a step further with their user friendly chatbot, ENO. In 2017, Capital One launched ENO, the world’s first natural language SMS based text assistant. What sets ENO apart is its ability to understand emojis and natural language, making it a breeze to have interactions with.
ENO uses something called a natural language processor to understand the different ways a human can talk about their money and their bank accounts. If you need to ask it about your bank balance, you don’t need to say the words, “What is my account balance?” Rather, you could just say, “How much money do I have?” or just send a money bag emoji either way it can answer your query. It has been trained to understand over 2,200 terms and emojis people use to ask about their account balances. Capital One’s AI team put in the hard work to ensure that ENO understands human nuances avoiding technical jargon all along.
“..creating the sophisticated NLP architecture to ensure ENO could understand nuances in human communication took an amazing amount of hard work creativity and collaboration..” -Margaret Mayer, the then vice president of software engineering at Capital One
How was ENO trained?
Firstly they pre-trained ENO with unsupervised learning techniques. It was trained with the chat generated from web chats between customer service representatives and real customers. It helped ENO to understand the similarities and attach meaning to the words generated within these conversations. Next it was trained with a supervised machine learning model using labeled data. It helped ENO to understand the tens of thousands of words and phrases customers had used previously. For example, when a customer wanted to activate a card he could have said, ‘Activate’, ‘turn on card’ or ‘make my card work.’
Finally an additional supervised learning was applied to new utterances so that ENO could understand terms that had been never used before. ENO not only recognizes similar terms and sentences but can also understand misspellings, abbreviations such as ‘pymt’ for payment, ‘Acct number’ for account number, hashtag (#) so on and so forth. And to add more to the human side of it, ENO also answers questions related to life and when that doesn’t work it can also crack jokes. Here’s the kicker, the team has also built a conversational context into ENO to make it feel like a friendly companion. It can pick up conversations right where you left off making interactions more human and engaging. So if you leave a conversation right in the middle for whatever reasons, don’t worry ENO understands! It can continue the chat being on the same page whenever you’re ready.
There you have it guys, AI is revolutionizing banking and finance industry in ways we couldn’t have imagined. From JP Morgan’s contract intelligence to PayPal’s fraud detection and capital One’s ENO chatbot the Innovations in AI and machine learning are enhancing efficiency, trust as well as customer experiences. And don’t let the fear of job losses overshadow the potential, as JP Morgan’s Chief Information Officer Dana Deasy wisely puts it, “AI is about freeing people to work on higher value tasks opening up new opportunities.” So as AI continues to evolve let’s embrace this transformative journey together.